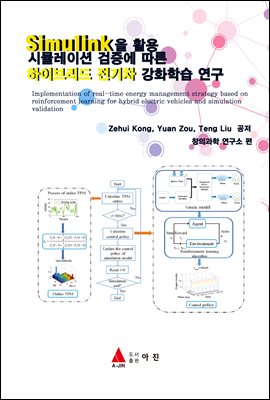
SimulinkÀ» Ȱ¿ë ½Ã¹Ä·¹ÀÌ¼Ç °ËÁõ¿¡ µû¸¥ ÇÏÀ̺긮µå Àü±âÂ÷ °ÈÇнÀ¿¬±¸
- ÀúÀÚZehui Kong, Yuan Zou, Teng Liu Àú
- ÃâÆÇ»ç¾ÆÁø
- ÃâÆÇÀÏ2020-07-13
- µî·ÏÀÏ2020-12-21
- SNS°øÀ¯
- ÆÄÀÏÆ÷¸ËPDF
- ÆÄÀÏÅ©±â12MB
- °ø±Þ»çYES24
-
Áö¿ø±â±â
PC
PHONE
TABLET
ÇÁ·Î±×·¥ ¼öµ¿¼³Ä¡
ÀüÀÚÃ¥ ÇÁ·Î±×·¥ ¼öµ¿¼³Ä¡ ¾È³»
¾ÆÀÌÆù, ¾ÆÀÌÆÐµå, ¾Èµå·ÎÀ̵åÆù, ÅÂºí¸´,
º¸À¯ 1, ´ëÃâ 0,
¿¹¾à 0, ´©Àû´ëÃâ 9, ´©Àû¿¹¾à 0
Ã¥¼Ò°³
To further improve the fuel economy of series hybrid electric tracked vehicles, areinforcement learning (RL)-based real-time energy management strategy is
developed in this paper. In order to utilize the statistical characteristics of online
driving schedule effectively, a recursive algorithm for the transition probability
matrix (TPM) of power-request is derived. The reinforcement learning (RL) is
applied to calculate and update the control policy at regular time, adapting to the
varying driving conditions. A facing-forward powertrain model is built in detail,
including the engine-generator model, battery model and vehicle dynamical model.
The robustness and adaptability of real-time energy management strategy are
validated through the comparison with the stationary control strategy based on
initial transition probability matrix (TPM) generated from a long naturalistic
driving cycle in the simulation.
Results indicate that proposed method has better fuel economy than stationary one
and is more effective in real-time control.
¸ñÂ÷
Á¦ 1Æí : SIMULINK ±âº»Æí1.1 SIMULINKÀÇ ½ÃÀÛ 1
ºí·ÏÀÇ ¿¬°á 5
ºí·Ï ÆÄ¶ó¹ÌÅÍÀÇ ¼³Á¤ 7
½Ã¹Ä·¹ÀÌ¼Ç ÆÄ¶ó¹ÌÅÍ (Configuration Parameters)ÀÇ ¼³Á¤ 8
½Ã¹Ä·¹À̼ÇÀÇ ¼öÇà 9
ºí·Ï ÆÄ¶ó¹ÌÅÍÀÇ Ç¥½Ã 9
º¹¼ö µ¥ÀÌÅÍÀÇ Ç¥½Ã 11
2.2 µ¿Àû ½Ã¹Ä·¹ÀÌ¼Ç 13
ÀÌÂ÷ ¹ÌºÐ¹æÁ¤½Ä 17
¼±Çü »óꝼö ¸ðµ¨ 23
DC ¸ðÅÍÀÇ ½Ã¹Ä·¹ÀÌ¼Ç 24
ÇÔ¼ö ºí·ÏÀÇ »ç¿ë 29
Â÷ºÐ¹æÁ¤½Ä(difference equation)ÀÇ ¸ðµ¨¸µ 34
Subsystem(ºÎ½Ã½ºÅÛ)ÀÇ ±¸¼º 37
Á¦ 2Æí : ¿¬±¸³í¹®
1. Introduction 41
2. Modelling of hybrid electric tracked vehicle 43
3. Real-time energy management strategy 45
4. RL-based Real-time energy management strategy 46
5. Simulation and validation 48
6. Conclusion 51
7. References 55