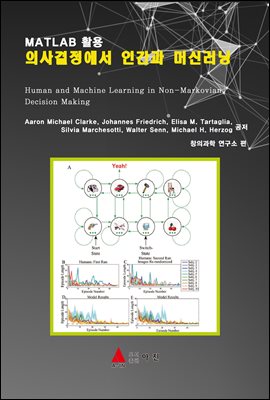
MATLABȰ¿ë ÀÇ»ç°áÁ¤¿¡¼ Àΰ£°ú ¸Ó½Å·¯´×
- ÀúÀÚAaron Michael Clarke, Johannes Friedrich, Elisa M. Tartaglia,Silvia Marchesotti, Walter Sen
- ÃâÆÇ»ç¾ÆÁø
- ÃâÆÇÀÏ2020-07-12
- µî·ÏÀÏ2020-12-21
- SNS°øÀ¯
- ÆÄÀÏÆ÷¸ËPDF
- ÆÄÀÏÅ©±â7MB
- °ø±Þ»çYES24
-
Áö¿ø±â±â
PC
PHONE
TABLET
ÇÁ·Î±×·¥ ¼öµ¿¼³Ä¡
ÀüÀÚÃ¥ ÇÁ·Î±×·¥ ¼öµ¿¼³Ä¡ ¾È³»
¾ÆÀÌÆù, ¾ÆÀÌÆÐµå, ¾Èµå·ÎÀ̵åÆù, ÅÂºí¸´,
º¸À¯ 1, ´ëÃâ 0,
¿¹¾à 0, ´©Àû´ëÃâ 6, ´©Àû¿¹¾à 0
Ã¥¼Ò°³
Humans can learn under a wide variety of feedback conditions. Reinforcementlearning(RL), where a series of rewarded decisions must be made, is a particularly
important type of learning. Computational and behavioral studies of RL have
focused mainly on Markovian decision processes, where the next state depends on
only the current state and action. Little is known about non-Markovian decision
making, where the next state depends on more than the current state and action.
Learning is non-Markovian, for example, when there is no unique mapping
between actions and feedback. We have produced a model based on spiking
neurons that can handle these non-Markovian conditions by performing policy
gradient descent [1]. Here, we examine the model¡¯s performance and compare it
with human learning and a Bayes optimal reference, which provides an
upper-bound on performance. We find that in all cases, our population of spiking
neurons model well-describes human performance.
¸ñÂ÷
Á¦ 1Æí : MATLAB ±âº»Æí1. MATLAB ±âº»»ç¿ëÆí ¡¤¡¤¡¤¡¤¡¤¡¤¡¤¡¤¡¤¡¤¡¤¡¤¡¤¡¤¡¤¡¤¡¤¡¤¡¤¡¤ 003
1.1 MATLAB ½ÃÀÛÇϱ⠡¤¡¤¡¤¡¤¡¤¡¤¡¤¡¤¡¤¡¤¡¤¡¤¡¤¡¤¡¤¡¤¡¤¡¤¡¤¡¤¡¤¡¤¡¤¡¤¡¤¡¤ 003
¸í·Éâ(command Window)¿¡¼ÀÇ ÀÔ·Â 005
µµ¿ò¸»(Help)ÀÇ ÀÌ¿ë ¡¤¡¤¡¤¡¤¡¤¡¤¡¤¡¤¡¤¡¤¡¤¡¤¡¤¡¤¡¤¡¤¡¤¡¤¡¤¡¤¡¤¡¤¡¤¡¤¡¤¡¤¡¤¡¤¡¤¡¤¡¤ 007
1.2 ÀÔ·Â ¿À·ùÀÇ ¼öÁ¤ ¡¤¡¤¡¤¡¤¡¤¡¤¡¤¡¤¡¤¡¤¡¤¡¤¡¤¡¤¡¤¡¤¡¤¡¤¡¤¡¤¡¤¡¤¡¤¡¤¡¤¡¤¡¤¡¤¡¤ 008
°è»êÀÇ ÁßÁö ¡¤¡¤¡¤¡¤¡¤¡¤¡¤¡¤¡¤¡¤¡¤¡¤¡¤¡¤¡¤¡¤¡¤¡¤¡¤¡¤¡¤¡¤¡¤¡¤¡¤¡¤¡¤¡¤¡¤¡¤¡¤¡¤¡¤¡¤¡¤¡¤¡¤¡¤¡¤¡¤¡¤¡¤¡¤¡¤¡¤¡¤ 009
MATLAB Á¾·áÇϱ⠡¤¡¤¡¤¡¤¡¤¡¤¡¤¡¤¡¤¡¤¡¤¡¤¡¤¡¤¡¤¡¤¡¤¡¤¡¤¡¤¡¤¡¤¡¤¡¤¡¤¡¤¡¤¡¤¡¤¡¤¡¤¡¤¡¤ 009
1.3 ¿¬»ê°ú º¯¼öÀÇ ÇÒ´ç ¡¤¡¤¡¤¡¤¡¤¡¤¡¤¡¤¡¤¡¤¡¤¡¤¡¤¡¤¡¤¡¤¡¤¡¤¡¤¡¤¡¤¡¤¡¤¡¤¡¤¡¤ 009
¿¬»êÀÚ ¿ì¼±¼øÀ§ ¡¤¡¤¡¤¡¤¡¤¡¤¡¤¡¤¡¤¡¤¡¤¡¤¡¤¡¤¡¤¡¤¡¤¡¤¡¤¡¤¡¤¡¤¡¤¡¤¡¤¡¤¡¤¡¤¡¤¡¤¡¤¡¤¡¤¡¤¡¤¡¤¡¤¡¤¡¤ 011
³»ÀåÇÔ¼ö ¡¤¡¤¡¤¡¤¡¤¡¤¡¤¡¤¡¤¡¤¡¤¡¤¡¤¡¤¡¤¡¤¡¤¡¤¡¤¡¤¡¤¡¤¡¤¡¤¡¤¡¤¡¤¡¤¡¤¡¤¡¤¡¤¡¤¡¤¡¤¡¤¡¤¡¤¡¤¡¤¡¤¡¤¡¤¡¤¡¤¡¤¡¤¡¤¡¤¡¤¡¤¡¤ 012
1.4 µ¥ÀÌÅÍÀÇ Ç¥Çö ¡¤¡¤¡¤¡¤¡¤¡¤¡¤¡¤¡¤¡¤¡¤¡¤¡¤¡¤¡¤¡¤¡¤¡¤¡¤¡¤¡¤¡¤¡¤¡¤¡¤¡¤¡¤¡¤¡¤¡¤¡¤¡¤¡¤¡¤¡¤ 013
1.5 º¯¼öÀÇ Ã³¸® ¡¤¡¤¡¤¡¤¡¤¡¤¡¤¡¤¡¤¡¤¡¤¡¤¡¤¡¤¡¤¡¤¡¤¡¤¡¤¡¤¡¤¡¤¡¤¡¤¡¤¡¤¡¤¡¤¡¤¡¤¡¤¡¤¡¤¡¤¡¤¡¤¡¤¡¤¡¤ 015
º¯¼ö À̸§ ¡¤¡¤¡¤¡¤¡¤¡¤¡¤¡¤¡¤¡¤¡¤¡¤¡¤¡¤¡¤¡¤¡¤¡¤¡¤¡¤¡¤¡¤¡¤¡¤¡¤¡¤¡¤¡¤¡¤¡¤¡¤¡¤¡¤¡¤¡¤¡¤¡¤¡¤¡¤¡¤¡¤¡¤¡¤¡¤¡¤¡¤¡¤¡¤¡¤¡¤ 015
clear ¸í·É¾î ¡¤¡¤¡¤¡¤¡¤¡¤¡¤¡¤¡¤¡¤¡¤¡¤¡¤¡¤¡¤¡¤¡¤¡¤¡¤¡¤¡¤¡¤¡¤¡¤¡¤¡¤¡¤¡¤¡¤¡¤¡¤¡¤¡¤¡¤¡¤¡¤¡¤¡¤¡¤¡¤¡¤¡¤¡¤¡¤¡¤ 016
Ư¼öº¯¼ö¿Í Á¤¼ö ¡¤¡¤¡¤¡¤¡¤¡¤¡¤¡¤¡¤¡¤¡¤¡¤¡¤¡¤¡¤¡¤¡¤¡¤¡¤¡¤¡¤¡¤¡¤¡¤¡¤¡¤¡¤¡¤¡¤¡¤¡¤¡¤¡¤¡¤¡¤¡¤¡¤¡¤¡¤ 017
whos ¸í·É¾î ¡¤¡¤¡¤¡¤¡¤¡¤¡¤¡¤¡¤¡¤¡¤¡¤¡¤¡¤¡¤¡¤¡¤¡¤¡¤¡¤¡¤¡¤¡¤¡¤¡¤¡¤¡¤¡¤¡¤¡¤¡¤¡¤¡¤¡¤¡¤¡¤¡¤¡¤¡¤¡¤¡¤¡¤¡¤¡¤ 017
1.6 º¤ÅÍ¿Í Çà·Ä ¡¤¡¤¡¤¡¤¡¤¡¤¡¤¡¤¡¤¡¤¡¤¡¤¡¤¡¤¡¤¡¤¡¤¡¤¡¤¡¤¡¤¡¤¡¤¡¤¡¤¡¤¡¤¡¤¡¤¡¤¡¤¡¤¡¤¡¤¡¤¡¤¡¤¡¤¡¤ 018
º¤ÅÍ ¡¤¡¤¡¤¡¤¡¤¡¤¡¤¡¤¡¤¡¤¡¤¡¤¡¤¡¤¡¤¡¤¡¤¡¤¡¤¡¤¡¤¡¤¡¤¡¤¡¤¡¤¡¤¡¤¡¤¡¤¡¤¡¤¡¤¡¤¡¤¡¤¡¤¡¤¡¤¡¤¡¤¡¤¡¤¡¤¡¤¡¤¡¤¡¤¡¤¡¤¡¤¡¤¡¤¡¤¡¤¡¤¡¤¡¤¡¤ 018
Çà·Ä ¡¤¡¤¡¤¡¤¡¤¡¤¡¤¡¤¡¤¡¤¡¤¡¤¡¤¡¤¡¤¡¤¡¤¡¤¡¤¡¤¡¤¡¤¡¤¡¤¡¤¡¤¡¤¡¤¡¤¡¤¡¤¡¤¡¤¡¤¡¤¡¤¡¤¡¤¡¤¡¤¡¤¡¤¡¤¡¤¡¤¡¤¡¤¡¤¡¤¡¤¡¤¡¤¡¤¡¤¡¤¡¤¡¤¡¤ 023
½ºÅ©¸° Ãâ·Â°ú ¾ïÁ¦ ¡¤¡¤¡¤¡¤¡¤¡¤¡¤¡¤¡¤¡¤¡¤¡¤¡¤¡¤¡¤¡¤¡¤¡¤¡¤¡¤¡¤¡¤¡¤¡¤¡¤¡¤¡¤¡¤¡¤¡¤¡¤¡¤¡¤ 024
1.7 ·£´ý(Random)¼ö¿Í º¹¼Ò¼ö ¡¤¡¤¡¤¡¤¡¤¡¤¡¤¡¤¡¤¡¤¡¤¡¤¡¤¡¤ 025
·£´ý ¼ö ¡¤¡¤¡¤¡¤¡¤¡¤¡¤¡¤¡¤¡¤¡¤¡¤¡¤¡¤¡¤¡¤¡¤¡¤¡¤¡¤¡¤¡¤¡¤¡¤¡¤¡¤¡¤¡¤¡¤¡¤¡¤¡¤¡¤¡¤¡¤¡¤¡¤¡¤¡¤¡¤¡¤¡¤¡¤¡¤¡¤¡¤¡¤¡¤¡¤¡¤¡¤¡¤¡¤ 025
º¹¼Ò¼ö ¡¤¡¤¡¤¡¤¡¤¡¤¡¤¡¤¡¤¡¤¡¤¡¤¡¤¡¤¡¤¡¤¡¤¡¤¡¤¡¤¡¤¡¤¡¤¡¤¡¤¡¤¡¤¡¤¡¤¡¤¡¤¡¤¡¤¡¤¡¤¡¤¡¤¡¤¡¤¡¤¡¤¡¤¡¤¡¤¡¤¡¤¡¤¡¤¡¤¡¤¡¤¡¤¡¤¡¤¡¤ 027
1.8 ±âÈ£¸¦ ÀÌ¿ëÇÑ ¿¬»ê ¡¤¡¤¡¤¡¤¡¤¡¤¡¤¡¤¡¤¡¤¡¤¡¤¡¤¡¤¡¤¡¤¡¤¡¤¡¤¡¤¡¤¡¤¡¤¡¤¡¤¡¤ 028
±âÈ£½Ä¿¡¼ÀÇ Ä¡È¯ ¡¤¡¤¡¤¡¤¡¤¡¤¡¤¡¤¡¤¡¤¡¤¡¤¡¤¡¤¡¤¡¤¡¤¡¤¡¤¡¤¡¤¡¤¡¤¡¤¡¤¡¤¡¤¡¤¡¤¡¤¡¤¡¤¡¤¡¤¡¤ 029
1.9 ÄÚµå ÆÄÀÏ ¡¤¡¤¡¤¡¤¡¤¡¤¡¤¡¤¡¤¡¤¡¤¡¤¡¤¡¤¡¤¡¤¡¤¡¤¡¤¡¤¡¤¡¤¡¤¡¤¡¤¡¤¡¤¡¤¡¤¡¤¡¤¡¤¡¤¡¤¡¤¡¤¡¤¡¤¡¤¡¤¡¤¡¤ 030
½ºÅ©¸³Æ® ÄÚµå ÆÄÀÏ ¡¤¡¤¡¤¡¤¡¤¡¤¡¤¡¤¡¤¡¤¡¤¡¤¡¤¡¤¡¤¡¤¡¤¡¤¡¤¡¤¡¤¡¤¡¤¡¤¡¤¡¤¡¤¡¤¡¤¡¤¡¤¡¤¡¤ 030
ÄÚ¸àÆ®ÀÇ Ãß°¡ ¡¤¡¤¡¤¡¤¡¤¡¤¡¤¡¤¡¤¡¤¡¤¡¤¡¤¡¤¡¤¡¤¡¤¡¤¡¤¡¤¡¤¡¤¡¤¡¤¡¤¡¤¡¤¡¤¡¤¡¤¡¤¡¤¡¤¡¤¡¤¡¤¡¤¡¤¡¤¡¤¡¤¡¤ 032
ÇÔ¼ö ÄÚµå ÆÄÀÏ ¡¤¡¤¡¤¡¤¡¤¡¤¡¤¡¤¡¤¡¤¡¤¡¤¡¤¡¤¡¤¡¤¡¤¡¤¡¤¡¤¡¤¡¤¡¤¡¤¡¤¡¤¡¤¡¤¡¤¡¤¡¤¡¤¡¤¡¤¡¤¡¤ 033
»ç¿ëÀÚ Á¤ÀÇÇÔ¼ö ¡¤¡¤¡¤¡¤¡¤¡¤¡¤¡¤¡¤¡¤¡¤¡¤¡¤¡¤¡¤¡¤¡¤¡¤¡¤¡¤¡¤¡¤¡¤¡¤¡¤¡¤¡¤¡¤¡¤¡¤¡¤¡¤¡¤¡¤¡¤¡¤¡¤¡¤ 036
1.10 °£´ÜÇÑ ±×·¡ÇÁÀÇ »ý¼º ¡¤¡¤¡¤¡¤¡¤¡¤¡¤¡¤¡¤¡¤¡¤¡¤¡¤¡¤¡¤¡¤¡¤¡¤¡¤¡¤¡¤ 037
ezplotÀ» ÀÌ¿ëÇÑ ±×·¡ÇÁ ¡¤¡¤¡¤¡¤¡¤¡¤¡¤¡¤¡¤¡¤¡¤¡¤¡¤¡¤¡¤¡¤¡¤¡¤¡¤¡¤¡¤¡¤¡¤¡¤¡¤¡¤ 037
plotÀ» ÀÌ¿ëÇÑ ±×·¡ÇÁ ¡¤¡¤¡¤¡¤¡¤¡¤¡¤¡¤¡¤¡¤¡¤¡¤¡¤¡¤¡¤¡¤¡¤¡¤¡¤¡¤¡¤¡¤¡¤¡¤¡¤¡¤ 039
3Â÷¿ø ±×·¡ÇÁ ¡¤¡¤¡¤¡¤¡¤¡¤¡¤¡¤¡¤¡¤¡¤¡¤¡¤¡¤¡¤¡¤¡¤¡¤¡¤¡¤¡¤¡¤¡¤¡¤¡¤¡¤¡¤¡¤¡¤¡¤¡¤¡¤¡¤¡¤¡¤¡¤¡¤¡¤¡¤¡¤¡¤¡¤¡¤ 042
1.11 MATLAB°ú ¿¢¼¿(Excel)ÀÇ Á¢¼Ó 043 ¿¢¼¿ µ¥ÀÌÅÍ ºÒ·¯¿À±â ¡¤¡¤¡¤¡¤¡¤¡¤¡¤¡¤¡¤¡¤¡¤¡¤¡¤¡¤¡¤¡¤¡¤¡¤¡¤¡¤¡¤¡¤¡¤¡¤¡¤¡¤¡¤¡¤¡¤ 043
µ¥ÀÌÅÍ °¡Á®¿À±â ¿É¼Ç ¡¤¡¤¡¤¡¤¡¤¡¤¡¤¡¤¡¤¡¤¡¤¡¤¡¤¡¤¡¤¡¤¡¤¡¤¡¤¡¤¡¤¡¤¡¤¡¤¡¤ 046
½ºÅ©¸³Æ® »ý¼º ¿É¼Ç ¡¤¡¤¡¤¡¤¡¤¡¤¡¤¡¤¡¤¡¤¡¤¡¤¡¤¡¤¡¤¡¤¡¤¡¤¡¤¡¤¡¤¡¤¡¤¡¤¡¤¡¤¡¤¡¤¡¤¡¤¡¤¡¤¡¤ 049
ÇÔ¼ö »ý¼º ¿É¼Ç ¡¤¡¤¡¤¡¤¡¤¡¤¡¤¡¤¡¤¡¤¡¤¡¤¡¤¡¤¡¤¡¤¡¤¡¤¡¤¡¤¡¤¡¤¡¤¡¤¡¤¡¤¡¤¡¤¡¤¡¤¡¤¡¤¡¤¡¤¡¤¡¤¡¤¡¤¡¤¡¤ 049
»ý¼ºµÈ µ¥ÀÌÅ͸¦ ¿¢¼¿ÆÄÀÏ·Î ÀúÀåÇϱ⠡¤¡¤ 050
Á¦ 2Æí : ¿¬±¸³í¹®
Human and Machine Learning in Non-Markovian Decision Making
1. Introduction 51
2. Results 53
3. Experiment 2: Intermixed Feedback 55
4. Modeling 56
5. Discussion 58
6. Materials and Methods 59
7. Procedures 60
8. Supporting Information 62
9. References 63